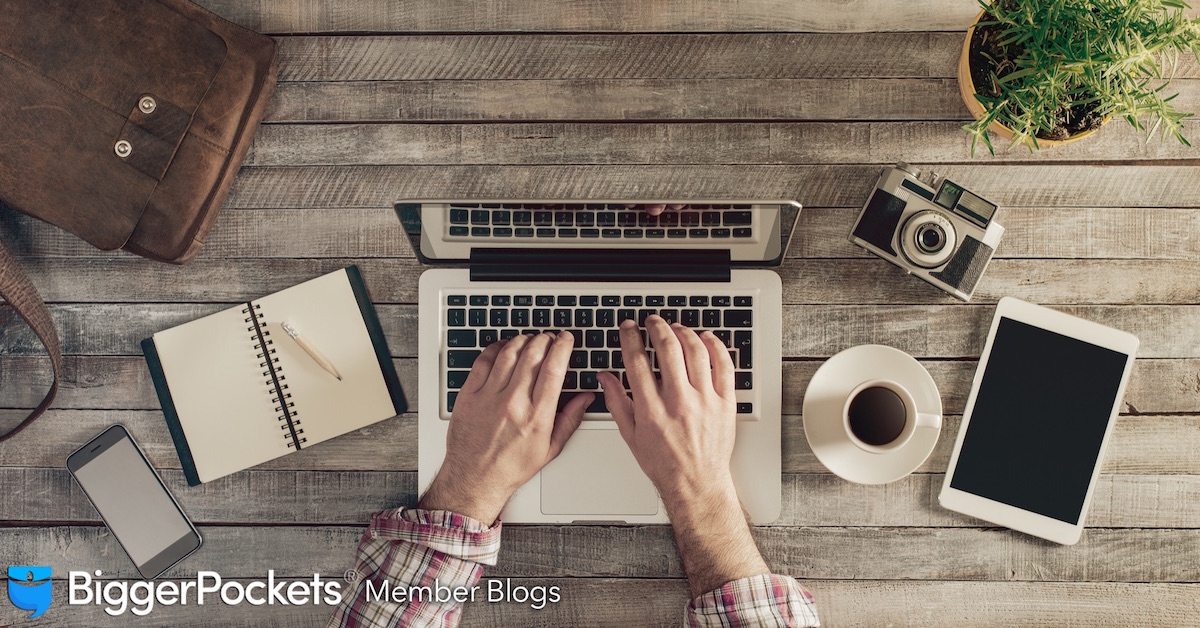

Thinking Outside the Black Box: Transparency in Real Estate Analytics
Many companies are emerging that use Artificial Intelligence to underwrite commercial real estate deals. In the quest for accuracy, this often involves the use of “ensemble models” or the averaging of many different models to improve accuracy.
No one disputes that accuracy is a good thing, but based on our customer feedback, transparency can be just as important. Accuracy can tell you what the price of a building may be, but transparency can tell you why. For example, if you’re developing a new multifamily property, a black box algorithm can tell you what your rents will be, but not the fact that you could’ve made your units 20% smaller and earned the same exact rents if you just put in common area barbecue grills and a dog run. In the real world, understanding what drives exceptional returns and how to optimize your execution can be more important than just predicting the end result.
With Enodo, we have pursued both accuracy and transparency in our models because at the end of the day people need to be able to understand and trust how they arrived at the given results. Here are two reasons transparency is even more important than accuracy in real estate predictive analytics:
You need consistent results that (generally) match with human intuition
Most real estate professionals have never trained a neural network on real estate data. That’s a given. If you had, then unless you have gigabytes of market and property data, you might be pretty surprised by the results. It might at first appear to predict very well – shockingly well actually. This would be particularly true if you tried to predict the data you trained on – when the training process is not properly managed, neural networks can easily “overfit” datasets, actually storing nearly EVERY variable used to make a prediction. This is like if you tried to predict the rent for every property in your database, by simply querying your database to get the rent.
This may sound great, but the truth is, if you try to apply the same model to a property outside of your database, the results could be very different from what you’d expect. Adding a bathroom to a unit could add something crazy like $1,500 in monthly rent, for example, or perhaps reducing square footage may actually increase rent. It’s difficult to know what is driving these changes and why – and you can’t rely on inconsistent results to underwrite real estate.
We experimented with models like this early on, and many real estate professionals did not appreciate the results. Unpredictable results are compounded when you are not careful about selecting features. Without human input on which features are important, AI can decide to use variables that don’t make any sense to real estate professionals. You could end up with something like Average Annual Rainfall being the biggest determinant of sale price. What? That wouldn’t make sense to any human being, and something that works in this way definitely shouldn’t be relied on to underwrite commercial real estate. Which brings us to the second point.
You need explainable results
The number one question real estate professionals ask after every demo (and one we actually have an answer for) is: “How did you come up with that number?” What this really means is: “How can I trust that number enough to use it in my analysis?” That’s a great question, and while traditional statistical models can answer it well, AI-based models typically can’t.
We’ve found that what is valuable in real estate decision making is understanding what can actually be done to improve investment performance. When you have a model that feeds variables into a black box and spits out a number, you have no idea which property or market characteristics drove performance and which ones hurt it. You have no idea how the different variables interacted, or which one was most important in determining value. You may get a prediction of rent or market value that looks spot on – but what does that really tell you?
The model thinks you’re right… that’s great. Now you can feel good that you’ve set rents correctly or paid the right price for an asset. That doesn’t tell you anything about what to do with that asset, or what to pay for the next one, however.
More importantly, though, if the AI is wrong, how would you know? Where did it weight a variable incorrectly? What data did it decide to leave out of the model? That’s scary. How can you invest millions, or tens of millions, or even hundreds of millions, without understanding how you arrived at the conclusion you did on valuation?
If you can’t explain how the model works and which variables are important, no one can or should trust your predictions. This is why at Enodo, we built a model that can explain the impact of each variable used in our predictions. Customers may see some variables or impacts that they don’t agree with for a particular market, but when it’s transparent, they can easily let us know, and we can improve the model. In this way, full transparency can provide valuable insight to both our customers and our data science team.
At the end of the day, AI is not magic and putting absolute trust into any black box model is probably a recipe for disaster. Accuracy is important, but transparency is even more important for facilitating trust, adoption and continual improvement.
Comments